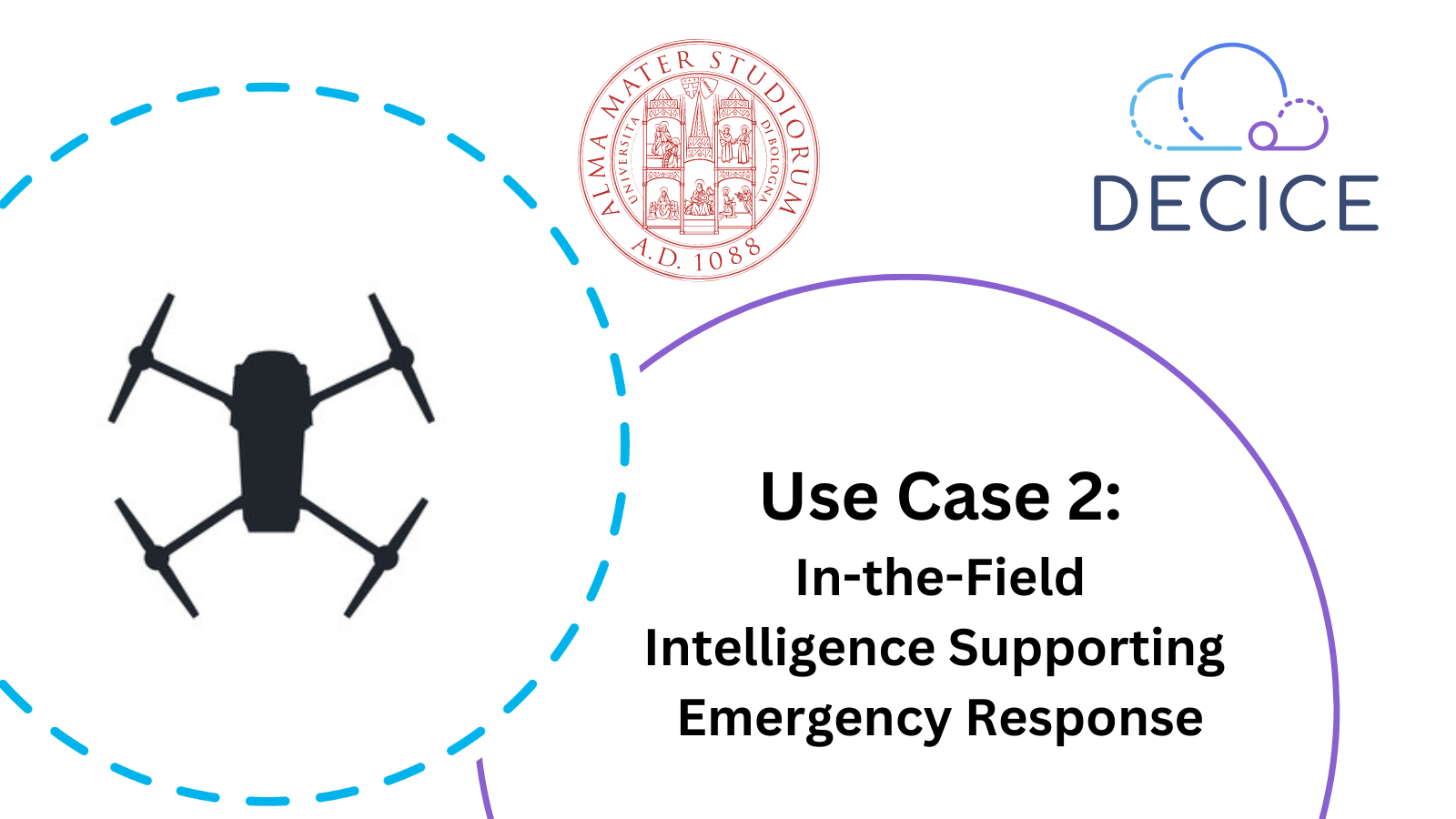
Use Case 2: In-the-Field Intelligence Supporting Emergency Response
Emergency response operations are pivotal in mitigating the impact of natural disasters, accidents, and other crises. Whether it’s earthquakes, floods, or humanitarian crises, the ability to locate and rescue individuals, acquire intelligence data from the field to support emergency responders and exploit robots in dangerous scenarios can save lives and lead to the success of the mission.
Our project aims to develop an open digital platform to enhance emergency response operators with data incoming from the field. The information is gathered with autonomous unmanned systems and in particular quadrotors. To manage this complex system made of many different computing units deployed in the field we will leverage the DECICE framework and its AI-based scheduler to effectively and energy-efficiently handle the management of the resources in the computing continuum.
The System
Our solution is composed of 3 different sub-systems: the cloud, the base station, and the autonomous drones. The objective is to bring intelligence to the operational activities in the field, by providing the computation support for embedding ML algorithms to support drone autonomous flight as well as mission operations. To do so, the platform will implement an efficient and adaptive computation of AI algorithms in the field, orchestrating the processing and the communication between the 3 subsystems. This will allow the operator to control the data flows between the computation entities and will provide adaptivity to the emergency scenario, such as the size of the area to be explored, recognition tasks to be performed, communication link bandwidth, and energy availability.
Autonomous drones can help to enable continuous monitoring of the affected areas and achieve an effective response after a disaster strikes.
Retrieved data from multiple drone onboard sensors (e.g., cameras and thermal cameras) and additional data sources such as satellite images can be integrated to define mission areas or processed to recognize and find survivors. This process can be executed onboard the drone or can be offloaded to the offboard computing units, depending on the AI scheduling operations carried out by the DECICE scheduler policy, which takes care of satisfying the constraints imposed by the use case.
Thanks to the support of machines, emergency responders do not risk their lives in dangerous search activities in dangerous areas.
To the best of our knowledge, there is no open software platform to coordinate emergency responders and autonomous drones during disasters. With this goal in mind, we aim to face all the technological challenges and unlock the possibilities described before by exploiting the new DECICE software stack to fill this gap.
Author: Sebastiano Mengozzi
Links
UNIBO: https://www.unibo.it/en
Keywords
Use Case | Drones | Cloud | Edge | In-field Devices | Emergency response
Links
AGENDA: Download NOW
SYNYO: https://www.synyo.com/
HUAWEI:
UGOE:
GWDG:
E4:
HLRS:
FBU:
TOP-IX:
UNIBO: https://www.unibo.it/en
MARUN: https://www.marmara.edu.tr/
BIGTRI: https://www.bigtri.net/
NAG: https://nag.com/
Keywords
Consortium | Event | Meeting | DECICE | Container | Kubernetes | KubeEdge | Hackathon | DECICE API | Digital Twin | AI models