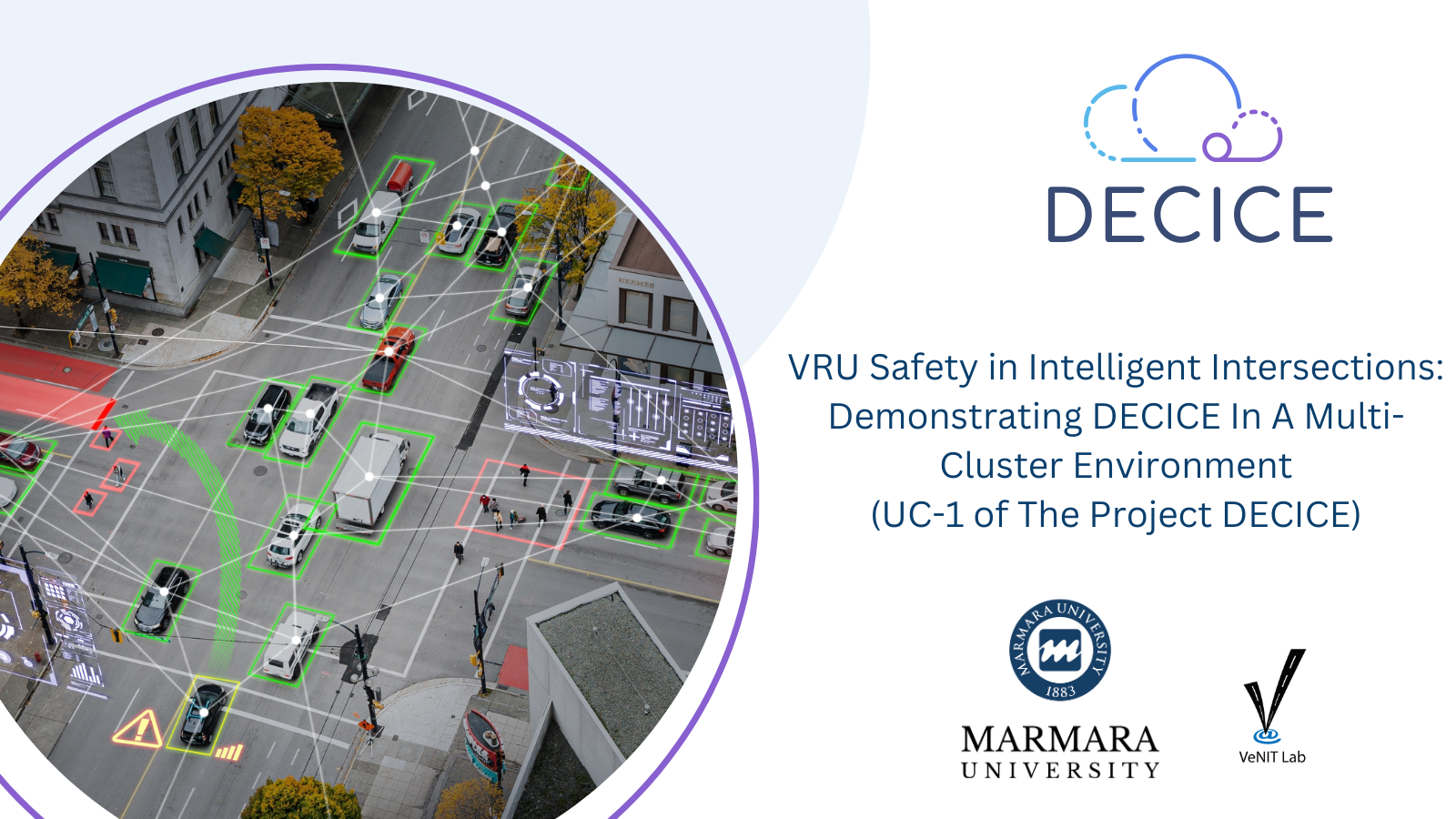
VRU Safety In Intelligent Intersections: Demonstrating DECICE In A Multi-Cluster Environment (UC-1 of The Project DECICE)
The Use Case 1 (UC-1), the first of three use cases in the DECICE project, is led by VeNIT Lab (Marmara University) and implemented by VeNIT Lab and BigTRI to enhance the safety of Vulnerable Road Users (VRU) at multiple intersections through real-time image processing and V2X communication. The system is now operating in a geographically distributed multi-cluster setup, collecting performance measurements in a heterogeneous cloud-edge environment. It demonstrates the edge-cloud continuum in action and provides insights for future network-aware scheduling within DECICE.
Real-Time Processing for Road Safety across Multiple Intersections
Designed for the Connected & Autonomous Vehicles (CAV) and Cooperative Intelligent Transport Systems (C-ITS) domains, this use case (UC-1) serves as a strong showcase of DECICE’s ability to efficiently schedule latency-sensitive workloads while optimizing energy consumption. In the use case, the inference workloads process real-time camera streams to detect and share information about vulnerable road users (VRUs) with nearby roadside units (RSUs) under strict latency and frequency requirements. To generate realistic C-ITS scenarios, the use case leverages Cilium Clustermesh for unified service discovery, cross-cluster workload communication, and federated monitoring of node, network, and inference/application metrics.
Heterogeneous Infrastructure with Real and Emulated Intersections
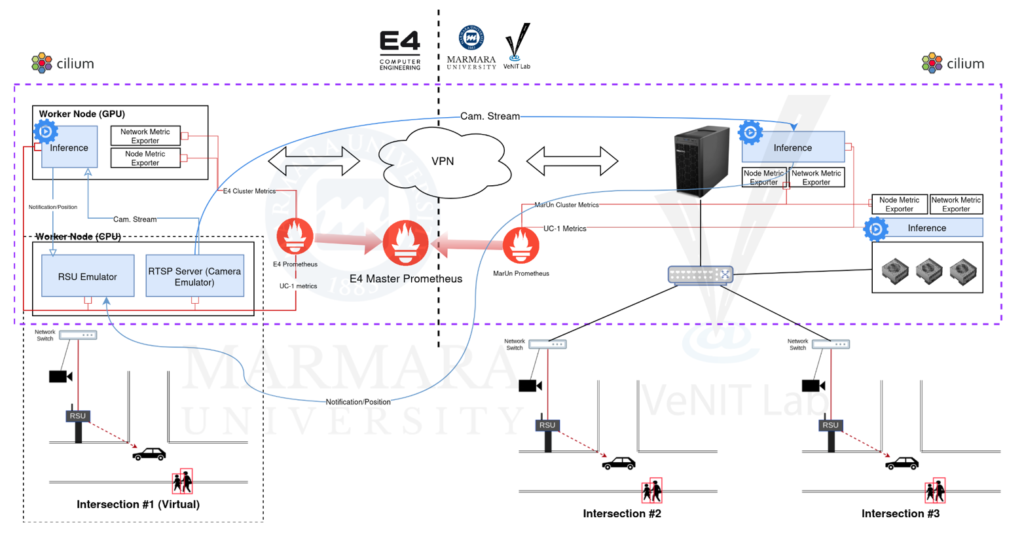
Figure 1: High-level architecture of the multi-cluster environment setup between VeNIT Lab (Marmara University) and E4
The demonstration of the use case operates on a setup where two geographically distributed Kubernetes clusters co-operate — one located at VeNIT Lab-Marmara University and the other at E4 Computer Engineering. These clusters are connected via Cilium Clustermesh and leverage KubeEdge for orchestration of edge nodes, integrating high-resource GPU nodes, ARM-based edge devices, and real or emulated roadside units(RSUs) and cameras. This setup forms the edge-cloud continuum and enables dynamic scenario generation by simulating intersections across multiple locations. It facilitates the discovery of inference applications, virtual intersections, and automated metric collection.
Real-time metrics related to edge node resource utilization, inference applications, and network conditions between edge nodes and devices across clusters are aggregated into a federated Prometheus instance. Network metrics, collected through WATMON Network Exporters (a software tool buılt by BigTRI), provide insights into node-to-node and node-to-device communication. These metrics are considered when testing different scenarios in the multicluster, such as assessing whether latency remains below 300 ms from the moment of capturing the camera frame to delivery of the image processing outputs (specifically the warnings or detected VRU spatiotemporal data) to RSUs for different workload placements across clusters.
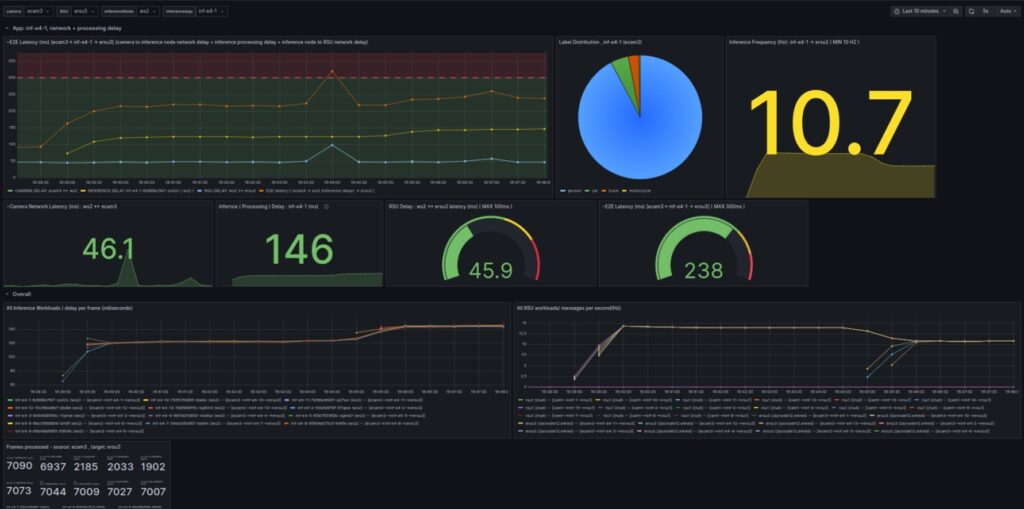
Figure 2: Grafana Dashboard for Intersection Metrics (by VeNIT Lab)
The collected metrics fed into DECICE’s Digital Twin, which represents in real-time the workloads, network, and node characteristics of the multi-cluster. This cluster snapshot is continuously recorded to a time-series database. The AI scheduler can leverage the historical or real-time data to make informed decisions, dynamically balancing latency, energy efficiency, and reliability for latency-critical workloads.
This use case (UC-1) in such a hybrid infrastructure serves as a strong demonstrator of DECICE’s ability to allocate and optimize resources, ensuring that latency-critical workloads are dynamically scheduled even under fluctuating conditions. The scheduling of the workloads in UC-1 will be adjusted based on energy, network, and performance requirements.
VeNIT Lab (Marmara University) and BigTRI will demonstrate, through this use case and the DECICE Framework, how heterogeneous infrastructures, digital twins, and AI-driven scheduling optimize real-time, edge-centric applications in the cloud-edge continuum.
Authors: Ozay Tokgozlu (VeNIT Lab), Berkay Yaman (BigTRI), Mujdat Soyturk (VeNIT Lab)
Keywords: Vulnerable Road User (VRU) detection, latency-critical, multicluster, heterogeneous cloud-edge, network-aware scheduling, Cilium Clustermesh, Kubeedge, Digital Twin, Prometheus, edge-cloud continuum.